Synthetic data: what is it and how is reshaping market research - a discussion with Hasdeep Sethi
Powered by Large Language Models (LLMs) and machine learning, synthetic data is rapidly emerging as a disruptive force in market research, promising to accelerate research processes, generate insights more quickly, and enhance decision-making. In this Q&A, Hasdeep Sethi, Data Science Director and AI Lead at STRAT7, discusses synthetic data’s role in market research, the impact it’s having on the industry, and the trade-off that needs to be made between speed and precision.
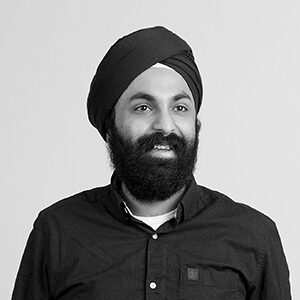
Hasdeep Sethi
Data Science Director and AI Lead at STRAT7
What is synthetic data?
Synthetic data, at its core, is artificially generated data that doesn’t originate from real human beings. It’s constructed either by predicting responses based on historical survey data or, increasingly, through advanced AI technologies like generative AI.
These AI models can be prompted with specific demographics and characteristics (e.g. age, gender, income) to simulate how a real person might respond to a survey question – rather than gathering data directly from human participants as we’d do in traditional research.
It’s important to distinguish between two key types of synthetic outputs:
-
Synthetic conversation tools (e.g. AI chatbots, personas):
These are built upon existing market research data, collected from real humans and allow users to interact with a simulated representation of a target audience. For example, you can use them to explore how different customer segments might respond to various marketing messages or product features. This gives you valuable insights into customer sentiment, without the time and expense of traditional research. -
Synthetic respondent data:
This involves generating entirely artificial responses, with little-to-no human input in the data collection process.
What are the main use cases of synthetic data in market research?
When trained on existing research, synthetic conversation tools can be used for market research in several ways. For example:
Concept testing:
Gauging the appeal of new product ideas across different customer segments.
Marketing and CRM optimisation:
Refining messaging and targeting for campaigns.
Product positioning:
Understanding customer pain points and designing products accordingly.
Predicting future behaviour:
Anticipating how different customer segments might respond to new offerings or changes in the market.
Synthetic respondent data is mainly used to supplement real survey samples. Researchers still survey real people, but synthetic data can boost representation in hard-to-reach demographics, like younger adults (18-24). This makes it particularly valuable when research requires dealing with smaller, less representative sub-groups.
What are some of the key benefits of using synthetic data in market research?
-
Reduced costs and accelerated research timelines:
Synthetic data can significantly lower research costs and speed up timelines by eliminating the need to recruit and survey real people at scale. -
Strengthened data quality and fewer gaps:
Synthetic data fills gaps in research samples, particularly for hard-to-reach demographics, and strengthens the quality of the information collected by augmenting existing datasets. -
Safe exploration of sensitive topics:
Synthetic data allows researchers to explore sensitive topics ethically and without compromising individual privacy, as it is not derived from real individuals. -
Consumer privacy and compliance assurance:
By not being linked to real individuals, synthetic data protects consumer privacy and helps businesses comply with data protection regulations. -
Agile testing and iteration:
The speed and cost-effectiveness of AI synthetic data generation make it ideal for rapid testing, iterative research and experimentation. -
Improved predictive modelling:
Synthetic data improves predictive modelling by providing large, diverse datasets for training and enhancing the accuracy of algorithms.
What are some of the key benefits of synthetic conversation tools like generative AI chatbots?
One of the main benefits of conversation tools is the ability to continually engage with their target audiences in a simulated environment. At STRAT7, we’ve built several segmentation chatbots that help clients “talk” to customer segments in natural language long after the initial research has been completed. That’s incredibly powerful. They can enhance and extend the value of that initial research – but – they shouldn’t be considered as a replacement for understanding your customers.
Another benefit is the ability to predict real human responses. In one study, we compared chatbot responses relating to new restaurant concepts with real survey data and found that the chatbot could predict group preferences with up to 90% accuracy.
Conversation tools can also reduce the need for frequent, smaller-scale surveys once a solid foundation of primary research exists, saving both time and money.
Can synthetic data ever fully replace real survey participants in market research?
We’re not there yet. It’s much faster to generate synthetic data than to gather human responses. But the trade-off is a decrease in the quality and precision of the data.
From what we’ve seen, synthetic samples often lack the consistency and precision of traditional research. For instance, they tend to exhibit less variation in their answers, clustering more towards the middle of response scales and being less likely to express strong opinions (e.g., “strongly agree” or “strongly disagree”). This can impact the quality and reliability of the research.
It’s also worth noting that different industries have varying risk appetites for synthetic data. For example, Pharma may be more cautious, as biases in generative AI models could lead to unreliable results, risking patient safety and regulatory non-compliance. When it comes to potential risks to human lives and health, it’s no surprise that there’s some nervousness out there!
What are some of the current disadvantages of synthetic data?
Despite its promise, synthetic data currently presents some key challenges. One significant issue is the potential for bias, as synthetic data models trained on biased datasets can perpetuate and amplify existing stereotypes. This is especially problematic for tasks like predicting consumer preferences or tailoring marketing messages.
Another challenge is the “black box” nature of some synthetic data tools, where the data generation process is opaque. This makes it difficult to verify the sources for the data and thus to trust the accuracy of the synthetic outputs. This lack of transparency can lead to “hallucinations,” where the model fabricates information.
Finally, data security is paramount. It’s crucial to ensure that synthetic data generation processes and the resulting datasets are handled securely, and in compliance with data privacy regulations to prevent misuse and protect sensitive information.
Read more – ‘A chatbot with principles. Eliminating key problems in the use of Generative AI in market research.‘
What steps can be taken to improve the depth, nuance, and human-like qualities of synthetic data?
For providers of synthetic respondent data, transparency is vital. They need to be open about the methodologies used to generate the data and disclose any potential biases related to demographics like gender or ethnicity. Transparency allows agencies like STRAT7 to evaluate the data and clearly communicate its limitations to clients. While real human data also contains biases, the nature of synthetic data generation process makes transparency a central consideration.
What more can we do to build trust in synthetic data outputs?
Insight teams and C-suite execs must communicate openly about synthetic data to establish a shared understanding of its strengths, limitations, and potential biases. This ensures everyone agrees on the level of weight to give synthetic data when informing key decisions. At STRAT7, we dedicate significant time to supporting our clients in developing internal trust.
For synthetic conversation tools, clear source information is crucial. Chatbots should provide citations for all data they use, so users can verify the information. This helps mitigate the risks of hallucinations or inaccuracies.
Another area to consider is synthetic media, like images and videos. As this field grows, watermarking synthetic content (e.g. Google’s SynthID) will become increasingly important, perhaps even a legal requirement, to easily distinguish it from real media.
How is synthetic data impacting the day jobs of insight professionals and researchers?
Synthetic data tools will likely require new skills. Designing deliverables to be interpreted easily by both humans and AI (chatbots) will be a major consideration. One impact of this may be the need to create different versions of reports – one for human consumption and another which is clearer for AI.
Prompt engineering – the art of effectively interacting with AI tools – will also be a crucial skill. As technology advances rapidly, researchers need to become skilled at creating the correct prompts to extract the desired information from these tools.
One of the goals of synthetic data is to free up researchers from manual tasks like questionnaire design and data entry, so they can focus on higher-value activities like strategic analysis and creative thinking. By automating routine tasks, synthetic data gives researchers more time to spend on generating actionable insights and contributing to strategic outcomes.
What does the future look like for synthetic data—where are we heading next?
The future of synthetic data looks promising. We can expect continued advancements in synthetic respondent data, but it remains to be seen whether it will ever reach the accuracy of human samples.
Adoption of synthetic tools, particularly client-specific chatbots, will grow, and we may see increased interest in synthetic qualitative data, such as AI-simulated in-depth interviews.
Find out more
Hasdeep Sethi is Director of Data Sciences at STRAT7 Bonamy Finch.
Get in touch to learn more about how STRAT7 can help you leverage the power of synthetic data.
Hasdeep Sethi
Data Science Director & STRAT7 AI Lead
STRAT7 Bonamy Finch
hasdeep.sethi@bonamyfinch.com